A/B Testing: The Key to Effective Healthcare Communications
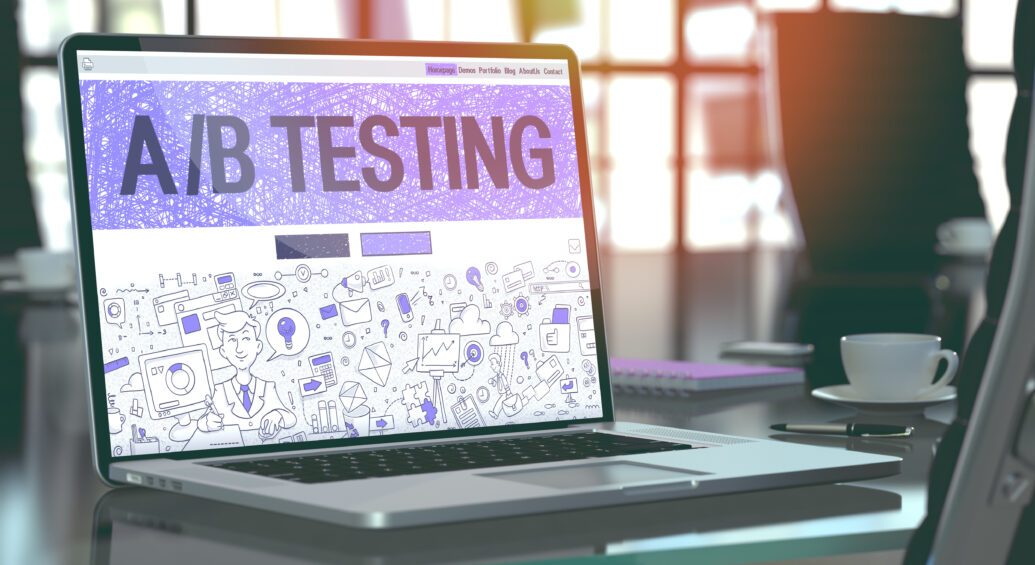
Changing behavior in healthcare is hard. How do we unlock the best way to motivate healthcare consumers when we are trying to reach and engage very diverse populations that include consumers from every walk of life? To complicate matters, we aren’t asking them to take just one action, we are asking them to take multiple, very personal actions related to their health. The answer to this question is A/B testing.
A/B (or A/B/n) testing is an experimentation methodology that compares two (A and B) or more (A through n) versions of a communication to determine which performs better. Leading companies in other industries like Microsoft, Amazon, and Google run more than 10,000 A/B tests every year that reach millions of consumers, according to Harvard Business Review. The healthcare industry can also harness of the power of A/B tests by testing different variations of the same communication to learn what works best.
Healthcare communications are necessary to inform your members of the services available to them, help them improve their health, and enhance the member experience. A/B testing is a way to make sure your efforts are as impactful as possible by continually iterating on “champion” versions to improve them. The following A/B testing guidelines are based on our experience with thousands of healthcare communication campaigns and tens of millions of member interactions.
Frequency
There’s no such thing as too much A/B testing in healthcare communications. The goal of A/B testing is to continually improve on the best results – whether those results are focused on increasing preventive exams, medication adherence, member satisfaction, or portal registrations. Any communication piece can benefit from experimentation, and all pieces should be viewed as opportunities to leverage this method. The process of A/B testing should be instilled as a companywide initiative and an overall mindset.
Variables
The beauty of A/B testing is that anything can be tested – as long as success can be measured. Testing communication channels is a great place to start and it works well with websites, member portals, live or automated calls, direct mail, email, social media ads, and text messages.
Many additional variables can be tested within each channel. Content, design elements, personalized content, and more can be tested in direct mail communications. Digital channels like email, websites, social media, and member portals can test myriad elements such as content in subject lines or message bodies, location or number of calls-to-action, time or day of delivery, imagery, or the frequency of messages or notifications. Channels can be tested together to determine the optimal modes, ordering, or cadence of omni-channel campaigns.
There are an infinite number of experiments that can be run to optimize any of your member communications, but your A/B test doesn’t end once you decide which variables you want to test. You must design your experiment properly in order to scale what you learn to future consumer outreaches.
Design
Good experiment design follows a methodology that can be easily replicated for any type of communications campaign. While there are many steps that go into a well-designed A/B test experiment, here are some top best practices to follow.
Begin with the end in mind. Even before considering what variables you want to test and how to test them, determine exactly what question you are trying to answer. For example, the question could be, “Does the text color of our call to action affect whether someone registers in our member portal?” or “Can we reduce the number of outbound calls without altering our program results?” The question should tie back to your strategy and serves as the foundation for your experiment hypothesis.
Establish your hypothesis. Your hypothesis is a statement – not a question – summarizing your informed opinion about the future outcome of your test. A hypothesis should be specific and testable. “A blue button will increase click rates more than a red button” is a valid hypothesis. “Which button will increase click rates?” is not.
Assign members to treatment groups. There are a couple of methods that are used most often* when assigning members to your test conditions: complete random sampling and stratified random sampling (randomizing members along select shared characteristics). Whichever method you choose, ensure that you are including a randomized control group. This can be the champion from a prior test or a complete hold-out group. In either case, it is important that members be randomized into the control group so as to avoid self-selection biases in your results. Work with your analytics team to determine how to choose an appropriate sample size based on the likely magnitude of impact.
*For those of you with some experiment design knowledge, I’m purposefully excluding multi-armed bandit algorithms despite some advantages in this space due to the relative complexity of operationalizing them.
Iterate quickly. Ensure that all of your members have the same timeframe or opportunity to act on your communication, and work with your analytics team to determine which statistical test is appropriate for your particular experiment design. Measure your results and apply the knowledge obtained to the next campaign quickly, rather than labor over a perfectly designed test that takes too long to get off the ground.
Insights
Through A/B testing, we have discovered many new best practices and overturned some previous standards. Sometimes the results fall perfectly in line with our hypotheses and other times previous champion approaches are determined to be ineffective – which is exactly why we encourage the use of continued A/B testing. Here are three insights from recent A/B tests in healthcare communications:
- Shorter messages tend to work better than longer messages at gaining attention – except when the longer message can succinctly communicate a benefit that the member values.
- Time of day does effect when members will answer phone calls – but the lift is not so substantial that it justifies adding additional operational complexity to a program.
- Adding a member’s name to an email subject line was a standard for driving much higher open rates in the past, but we have discovered that this trend has reversed in recent years as the approach is now closely associated with spam messages.
Including A/B testing into your healthcare communications can dramatically improve the results of your campaigns leading to better health outcomes, improved member experience, and lower costs. The true winners of any continuous A/B testing program are your members.